[Column] Mobile Lending – Non-traditional data in credit decision making
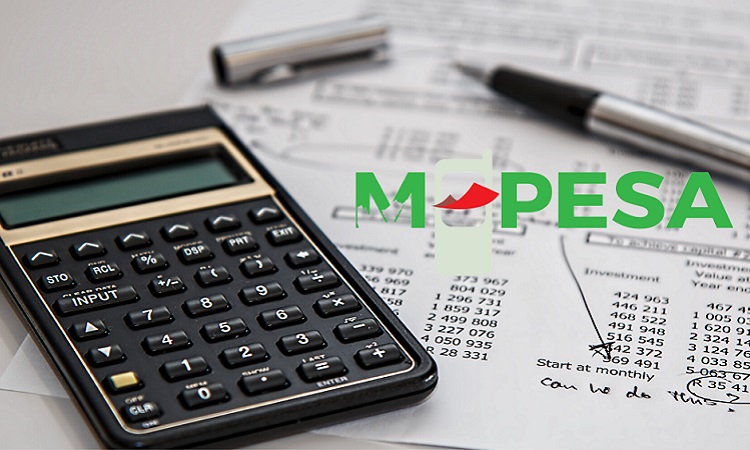
Retail lending in Kenya has taken a unique path. It has skipped some stages typical for developed markets and climbed to heights that can be admired and envied. Ironically, this happened not due to economical advantages but rather due to certain historical deficiencies.
As it happens in many developing markets at some point lots of people move from rural areas to big cities seeking better-payed jobs. These people as they earned money needed some way to transfer it to their families, often left in the villages. Most of these people didn’t have a banking account as banks were avoiding such customers, viewing them as high risk/low profit. In such circumstances a person wishing to transfer money to his family would have to find a bus driver, going to his home village, and ask him to carry cash to his family. Not the most fast, convenient and secure way to commit a financial transaction.
In 2007 Safaricom, a Kenyan telecom company addressed this problem by launching MPESA – mobile wallet linked to user’s phone number. A user could send money to another person over network who could then withdraw funds from the system through an agent. The agents were either Safaricom’s employees or shopkeepers who partnered with Safaricom besides of doing their primary business. With 93% of population coverage by mobile (Safaricom’s Annual Report 2019) and ubiquitous agents network the problem of money transfers was solved. People started to use MPESA to transact with each other, and also with businesses and government agencies. Since 2007 mobile money has rapidly proliferated in the developing world and today there are more than 500 MLN users in at least 90 countries (GSMA 2016a).
Besides solving money transfer problem MPESA has propelled the development of retail lending. Millions of people that hadn’t had access to finance suddenly entered the financial system. They sent money to each other, payed bills and purchased goods through mobile wallet. Whereas many people in Kenya are not officially employed (according to the National Bureau of Statistics informal sector represents 82,7% of employment) this data is the only reliable footprint of their financial activity.
Mobile lending has become a critical growth driver of lending market. A typical mobile lender is a financial institution partnering with mobile operator (e.g. Commercial Bank of Africa partnered with Safaricom and launched their M-Shwari product in 2012). A credit application is submitted through mobile phone (app or USSD interface) and remotely processed by Lender. In case of positive decision money are funded on person’s mobile wallet. Mobile wallet is also used to make regular loan payments. Such purely digital approach has obvious benefits. Since loan is disbursed remotely Lender doesn’t have to extend sales network, keeping operational costs low. The disbursement is made immediately without need to visit bank’s office which improved customer experience and boosted growth. The weak sides of such approach are limited KYC (which is basically delegated to a telecom company) and weak collection (in most cases customers are out of physical reach of Lender). This results in higher than usual NPL rate which is compensated by higher interest rate (or service charge).
At the dawn of mobile lending credit limits were relatively small, starting from 500 KSH (5 USD). Now, banks are ready to provide 50,000 KSH (500 USD) and even higher amounts which is on par with traditional unsecured loan. This has become possible due to building up of good customer base and advanced scoring mechanisms that rely on a vast array of data, both traditional (e.g. credit bureau) and non-traditional (e.g. phone meta-data, mobile wallet). Non-traditional data proved to be useful for assessing customers without previous credit history (64% according to The World Bank).
Data sources include calls journal, installed apps, SMS and other. Most of such data is not linked to credit behavior but some subtle items matter a lot. For example, Creditinfo has conducted research that showed that customers who have installed UBER app are more reliable than those without. Gambling apps, on the other hand, indicate higher risk. Another research showed that people who have certain government agencies in their phone book are less risky than the rest.
In the domain of non-traditional data mobile wallet proved to be the most powerful resource. Data on financial transactions is used to estimate customer’s income and to assign credit limit. A person that is regularly paying 5,000 KSH bill is likely to have enough income to serve 10,000 KSH loan given for three months. Of course, different people have different financial behavior so designing limit assignment system is not a straightforward exercise and requires solid analytical work. The advanced approach recommended by Creditinfo’s consultants combines specific MPESA variables with an internal/CRB score breaking down population by customer segments (e.g. Prime/Sub-prime). Higher limits are provided to low risk/high income customers which leads to increased profitability and reduces risk exposure. A good limit assignment system will also consider length of relation with customer and his borrowing activity. New customers will typically get smaller amounts than those who have already repaid several loans. This strengthens customer relations and helps building up good customer base. Implementation of such advanced approach requires besides of heavy analytical work availability of proper automation tools that support not only scorecard calculations but also complicated decision matrices varying by customer segments. Furthermore, as every analytical model limit assignment model requires regular reviews and fine-tuning. We need to monitor stability of financial behavior patterns and alignment of limit size with level of risk. It becomes possible only if historical decision data is properly stored and maintained, and can be easily linked to customer’s payment behavior.
We are yet to observe what will happen to lending market in Kenya. Recently the growth has slowed down which might indicate a tipping point in the credit cycle, followed by a decline and growth of NPL. Still the lessons learned are insightful and can be utilized in other developing markets.
This column was submitted by Maxim Fetisov, the Global Consultant at Creditinfo Group.
Follow us on Telegram, Twitter, Facebook, or subscribe to our weekly newsletter to ensure you don’t miss out on any future updates. Email your columns to info@techtrendske.co.ke